The concept of precision versus accuracy comes up across many industries, and is ultimately the same set of principles used in relation to data and how it is used.
Ray already touched on this in his post on qualitative and quantitative risk analysis, when he says it is ‘better to be shotgun accurate than stormtrooper precise’, but I thought I’d spend a bit more time reflecting on the whole issue of precision versus accuracy and why you should probably give it some thought when it comes to risk assessments.
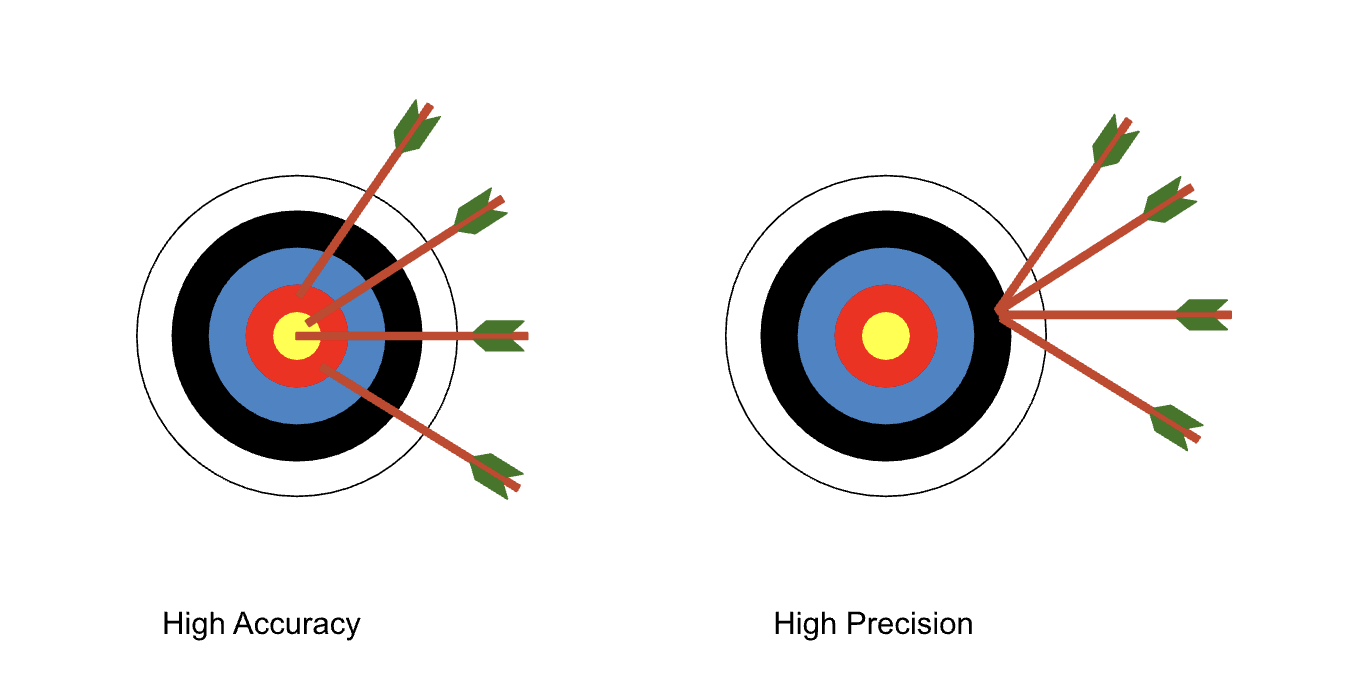
Accuracy
Accuracy is all about the degree to which the data values chosen reflect their real/true values. So when it comes to coming up with estimates, this reflects our capability to provide correct information.
Precision
Precision is a measure of how much confidence you have in your analysis and/or inputs and as a result, how exact you can be in your estimates.
🎂
So if you asked how much of a cake has been eaten, “63.56%” would be a precise answer but “two thirds” might be a more accurate one".
So which is better?
When it comes to making estimates for the purposes of risk analysis, accuracy is generally much more important than precision. It is important to keep in mind that our goal with quantitative risk analysis is reducing uncertainty, expressed as a probable loss exposure; we are not making a prediction so we don’t need to be precise! Let’s explore why in a bit more detail.
The results of any quantitative analysis are always presented in ranges to account for the uncertainty of the risk assessment and not surprisingly, the inputs are also represented as a range of values. When working with our clients to estimate probability values, the aim is to come up with lower and upper bounds that define a 90% confidence level, where 9 times out of 10, the client is happy that the actual value will fall between these two bounds.
Using ranges like this can bring higher degrees of accuracy to estimates (90% within the range being the aim), with a precision based on how confident we are in our estimates. If we don’t have much data to inform our estimates, or the data we do have shows a wider variance, our 90% confidence bounds are going to be quite wide. If you have lots of data to inform your analysis, you’re going to have much narrower bands of error and your 90% confidence levels will be much closer in.
Using ranges also helps account for uncertainty by dealing with peoples’ estimates that are precise but entirely wrong. If a qualitative risk assessment says there is a 90% chance that a risk scenario occurs, you have to ask yourself: what is being achieved by being so precise, if the number is completely inaccurate?
“If we can’t be precise, what’s the point?”
Coming up with a range of possible values can seem counterintuitive when you first start. The problem is that traditional approaches to qualitative risk assessments give an illusion of precision. A 1-5 rating for probability and/or impact is chosen to infer a level of numerical precision, but where did this number come from? It invariably came from a lookup table where a value of 3 for probability is allowed for anything between let’s say 75% to 90% (which will have a label something like ‘highly probable’). So the risk assessor wasn’t being any more precise with their analysis, but perhaps more importantly didn’t get to choose their 90% confidence level. They were forced into picking from pre-defined boxes, which may only be actually correct 50% of the time! The qualitative analysis ends up being overly precise, at the expense of accuracy.
To put it in more practical terms: consider the possible range of costs for a ransomware attack. That figure could be anything from £10 to £10 million. If you’re the person using the cyber risk register to make strategic investment decisions, you’d probably rather know that your likely losses will fall within a particular range, than be a predicted exact figure.
Accuracy is a great thing to aim for - but let precision be guided by your confidence. Or lack of it.
Headline photo by Ricardo Arce on Unsplash